Predictive analytics in AI involves using advanced algorithms and statistical models to analyze historical data and identify patterns, trends, and potential future outcomes. It leverages machine learning techniques to make predictions and forecasts, enabling businesses and organizations to anticipate future events or behaviors. By utilizing data-driven insights, predictive analytics helps in decision-making, risk assessment, and strategic planning. This powerful application of artificial intelligence enables proactive decision-making and enhances overall efficiency across various industries. Explore how predictive analytics can transform your business by harnessing the power of AI to foresee and prepare for what lies ahead.
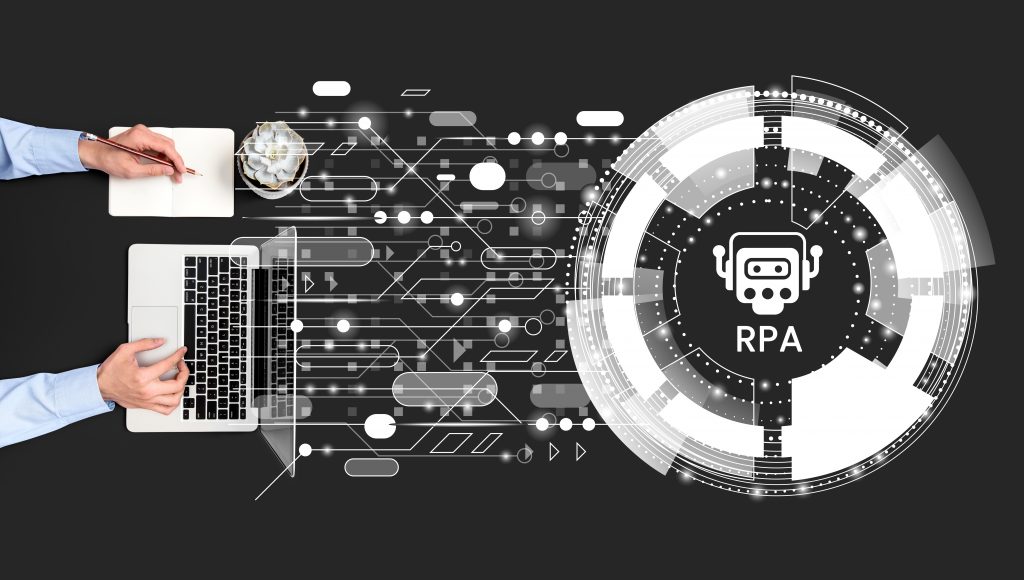
In the ever-evolving landscape of Artificial Intelligence applications, organizations are leveraging advanced analytics and data mining techniques to facilitate data-driven decision-making. At the forefront of this transformative journey are using predictive analytics tools, reshaping the way businesses operate and strategize in diverse industries. Understanding the significance of data-driven decision-making, businesses now embrace sophisticated data mining techniques and advanced analytics to gain actionable insights.
This shift towards informed decision-making is fueled by the integration of artificial intelligence applications, where in using predictive analytics in AI tools play a pivotal role. Predictive analytics, as a subset of advanced analytics, empowers organizations to forecast future trends, behaviors, and outcomes. This blog post aims to provide a comprehensive guide to the transformative power of predictive analytics in AI across industries. We will explore its core principles, delve into real-world applications in finance, healthcare, marketing, and more, and navigate the strategic process involved in implementing these data-driven solutions.
As businesses grapple with the challenges of an increasingly data-centric landscape, understanding and harnessing the capabilities of predictive analytics tools becomes crucial. This post will shed light on overcoming challenges, optimizing the strategic predictive analytics process, and offer insights into future trends that are shaping the data-driven landscape. Join us on this journey through the realms of data mining techniques, data-driven decision-making, artificial intelligence applications, advanced analytics, and the indispensable role of predictive analytics tools in steering businesses towards a more insightful and competitive future. Join us on this journey through the realms of data mining techniques, data-driven decision-making, artificial intelligence applications, advanced analytics, and the indispensable role of predictive analytics tools in steering businesses towards a more insightful and competitive future.
At its core, predictive analytics represents a leap into the future, Predictive Analytics Tools where historical data, statistical algorithms, and machine learning converge to provide insights into forthcoming outcomes. Unlike traditional analytics that primarily focuses on retrospective analysis, predictive analytics empowers organizations to anticipate trends and make informed decisions proactively. Predictive analytics is the branch of advanced analytics that utilizes data, statistical algorithms, and machine learning techniques to identify the likelihood of future outcomes based on historical data. In simpler terms, it involves extracting meaningful patterns and trends from past information to make informed predictions about what might happen in the future.
The Core Components in Predictive Analytics in AI: Data, Algorithms, and Machine Learning
- Data as the Foundation:
Predictive analytics stands on the bedrock of data – the lifeblood of AI (Artificial Intelligence Applications). The richness, diversity, and accuracy of the dataset directly influence the efficacy of predictions. From unraveling customer behavior patterns to discerning market trends, Data Science Strategies predictive analytics is advanced analytics excels in extracting meaningful insights from vast and varied datasets.
- Algorithms & Machine Learning’s Role:
Driving the predictive analytics engine are algorithms – intricate mathematical models that sift through data to identify patterns, correlations, and trends. As technology advances, these algorithms evolve, becoming more sophisticated and adept at handling increasingly complex datasets. Machine learning serves as the powerhouse behind predictive analytics. Through iterative learning, machines continually enhance their performance, adapting to new data and refining predictions. This dynamic process significantly augments the accuracy and reliability of predictive models over time.
Applications across Industries
- Financial Sector & Healthcare Revolution:
In the financial realm, predictive analytics plays a pivotal role in risk management. Analyzing historical market data, economic indicators, and even social media sentiment enables financial institutions to make informed decisions about investments, identify potential fraud, and optimize portfolio management. Predictive analytics has become a linchpin in the healthcare sector, from predicting disease outbreaks to personalizing treatment plans based on patient data. This transformative application not only improves patient outcomes but also optimizes resource allocation within healthcare systems.
- Marketing Precision & Supply Chain Optimization:
Marketers harness the power of predictive analytics to tailor strategies with laser precision. By analyzing customer behavior, preferences, and purchase history, businesses can anticipate future trends, optimize marketing campaigns, and elevate overall customer satisfaction. In logistics and supply chain management, predictive analytics serves as a cornerstone for optimizing inventory levels, predicting demand fluctuations, and streamlining logistics operations. This not only reduces costs but also ensures a smoother and more responsive supply chain.
- Human Resources Planning:
Predictive analytics transforms human resources management, from talent acquisition to employee retention. Analyzing data on employee performance, satisfaction, and turnover empowers organizations to make informed decisions about hiring, training, and talent management.
The Predictive Analytics in AI Process: Navigating a Strategic Journey
- Define Objectives:
Embarking on a successful predictive analytics journey begins with a crystal-clear definition of objectives. This strategic planning phase lays the foundation for the entire process, outlining specific goals such as reducing customer churn, enhancing sales forecasts, or optimizing resource allocation. A well-defined objective acts as a guiding beacon throughout the predictive analytics journey.
- Data Collection & Data Cleaning and Pr-processing:
The heart of predictive analytics lies in data processing. Big data analytics is robust and diverse datasets are essential for accurate predictions. During this phase, data is gathered from various sources, including historical records, customer interactions, and social media sentiment. The richness of the dataset directly influences the model’s ability to uncover meaningful insights. Data quality is paramount in predictive analytics in data-driven decision making. Before analysis commences, collected data undergoes rigorous cleaning and per-processing to rectify inconsistencies, errors, or missing values. The integrity of the data is crucial, as the predictive model’s accuracy hinges on the quality of the input and data mining techniques.
- Feature Selection & Choosing the Model:
Not all data is created equal. Feature selection involves identifying the most relevant variables that significantly influence the predictive model. Selecting the right features enhances the model’s efficiency and accuracy, focusing on key factors driving outcomes. Selecting an appropriate predictive model is a pivotal decision. Depending on the nature of the data and objectives, various models such as linear regression, decision trees, and neural networks may be considered. The choice of model shapes the analytical approach and influences predictive capabilities.
- Training the Model & Evaluation and Validation:
Training the model is a dynamic process where the selected algorithm learns from historical data. Through iterative learning, the model adapts and refines predictions, improving performance over time. The effectiveness of this training phase is crucial for accurate predictions based on data patterns. Once trained, the model undergoes rigorous evaluation and validation using separate datasets. This step ensures the model performs reliably and consistently. Evaluations metrics help assess accuracy, and any adjustments needed for optimization are identified during this phase.
- Deployment & Monitoring and Maintenance:
With a validated and optimized model, the deployment phase involves integrating it into operational environments. This could include incorporating the model into existing systems, software, or platforms, allowing organizations to leverage its predictive capabilities in real-world scenarios. Implementation of predictive analytics doesn’t end with deployment. Continuous monitoring of the model’s performance is essential. On-going assessment ensures accuracy and relevance as data evolves. Regular maintenance and updates adapt to changing environments and emerging patterns.
Overcoming Challenges in Predictive Analytics In AI: Navigating a Data-Driven Landscape
- Data Quality & Data Privacy and Security:
Ensuring data quality is a perpetual challenge. Incomplete, inaccurate, or biased data can lead to flawed insights and unreliable predictions. Organizations invest in robust data governance practices, data cleansing tools, and validation protocols to address and overcome data quality issues. As reliance on data intensifies, safeguarding data privacy and security is paramount. Predictive analytics often involves handling sensitive information, necessitating stringent security measures, encryption protocols, and compliance with data protection regulations to protect against breaches and unauthorized access.
- Interpret-able Models & Continuous Learning:
The interpret-ability of predictive models, especially complex ones like neural networks, is a challenge. Understanding how a model arrives at specific predictions is crucial for building trust and ensuring accountability. Developments in explainable AI aim to make complex models more transparent and interpret-able. Predictive models need to adapt to changing environments and evolving data. Continuous learning mechanisms, such as updating models with new data and re-calibrating algorithms, are essential to maintaining relevance and accuracy over time. This proactive approach ensures predictive analytics remains effective in dynamic scenarios.
The Future Landscape of Predictive Analytics: Pioneering New Frontiers
- Explainable AI & Automated Machine Learning (AutoML):
The evolution of predictive analytics includes a growing emphasis on explainable AI. This trend aims to make complex models more transparent and understandable. Users, including business stakeholders and decision-makers, benefit from a clearer understanding of how the model generates specific predictions, fostering trust and confidence in AI-driven decision-making. The democratization of predictive analytics is on the horizon with the rise of Automated Machine Learning (AutoML). AutoML streamlines the entire analytics process, automating tasks from data preparation to model deployment in AI-driven Insights. This democratization makes predictive analytics more accessible, reducing barriers for aspiring data scientists and analysts.
- AI-powered BI & Edge Computing for Predictive Analytics in AI :
The integration of AI into business intelligence (BI) tools is reshaping decision-making. AI-driven BI platforms provide real-time insights, empowering organizations to make data-driven decisions swiftly and accurately. This integration enhances the speed and efficiency of decision-making processes across various sectors. Edge computing is emerging as a transformative trend in predictive analytics. By bringing analytics closer to the data source, edge computing reduces latency and enables real-time predictions. This is particularly valuable in scenarios where immediate decision-making is critical, such as in IoT (Internet of Things) applications and industries requiring instant responses.
Conclusion: Navigating a Data-Driven Future
In conclusion, the predictive analytics process is a multifaceted journey involving meticulous planning, data preparation, model training, and on-going optimization. Organizations can unlock the full potential of predictive analytics by proactively addressing challenges related to data quality, privacy, model interpret-ability, and continuous learning. Looking ahead, the landscape of predictive analytics holds exciting prospects with explainable AI, Automated Machine Learning, AI-powered Business Intelligence, and the integration of edge computing. Embrace the potential of predictive analytics to chart a course toward innovative, informed, and empowered decision-making in the ever-evolving world of AI.